Working with missing values is a common task in machine learning. We can say that it’s the very first task to accomplish before starting a pre-processing pipeline. The most common approach to blank filling is to use the mean and the median values. Although this is a very common practice, maybe there’s a more data-driven approach we can use.
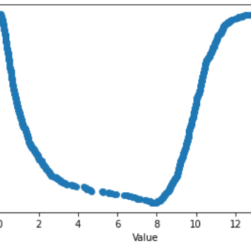